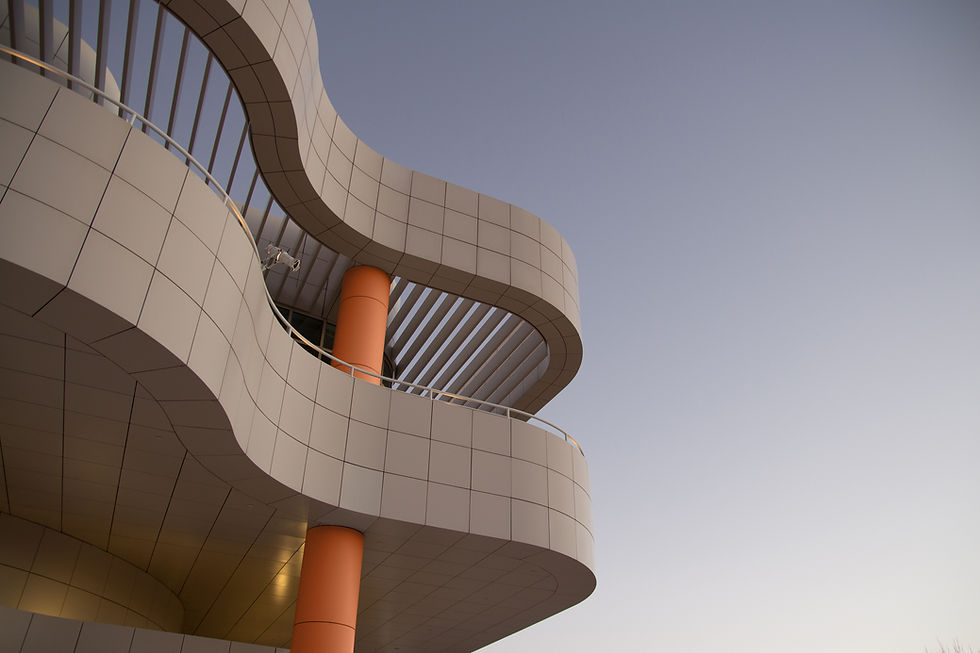
Understanding the Basics of Inferential Statistics & Hypothesis Testing
May 16, 2024
5 min read
0
12
In today's data-driven world, understanding inferential statistics and hypothesis testing is crucial for making informed decisions based on data analysis. Whether you're a researcher, analyst, or business professional, grasping these concepts empowers you to draw meaningful conclusions from data and make predictions about populations based on sample data.

Introduction to Inferential Statistics
What is inferential statistics?
Inferential statistics entails drawing conclusions or making predictions about a population by analyzing a sample of data. This process enables us to extend our findings from a sample to a broader population.
Importance of inferential statistics
Inferential statistics enables us to draw conclusions, make predictions, and test hypotheses about populations, which is essential for scientific research, business analytics, and decision-making.
Population vs. Sample
Understanding population
A population denotes the complete set of individuals or objects that pique our interest for study.
Definition of a sample
A sample is a subset of the population that is selected for observation and analysis.
Importance of sampling in inferential statistics
Sampling allows us to gather data efficiently and cost-effectively, providing insights into the characteristics of the larger population.
Probability Distribution
Definition of probability distribution
A probability distribution describes the likelihood of different outcomes in a statistical experiment.
Types of probability distributions
Common probability distributions include the normal distribution, binomial distribution, and Poisson distribution.
Role of probability distribution in inferential statistics
Probability distributions serve as the basis for making probabilistic statements and conducting hypothesis tests.
Central Limit Theorem
Explanation of the central limit theorem
The central limit theorem asserts that as the size of the sample increases, the distribution of sample means tends to resemble a normal distribution, regardless of the original shape of the population distribution.
Importance of the central limit theorem in inferential statistics
The central limit theorem allows us to make inferences about population parameters using sample statistics, even when the population distribution is unknown.
Hypothesis Testing: Basics
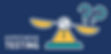
What is hypothesis testing?
Hypothesis testing is a statistical method used to make inferences about a population parameter based on sample data.
Importance of hypothesis testing in inferential statistics
Hypothesis testing allows us to evaluate theories, test assumptions, and make decisions in the face of uncertainty.
Steps in Hypothesis Testing
Formulating null and alternative hypotheses
The null hypothesis (H0) states that there is no significant difference or effect, while the alternative hypothesis (Ha) suggests otherwise.
Choosing a significance level
The significance level (alpha) determines the threshold for rejecting the null hypothesis.
Conducting the test
Based on the sample data, we calculate a test statistic and compare it to a critical value or p-value to determine the statistical significance.
Interpreting the results
We interpret the results of the hypothesis test based on the calculated p-value and the chosen significance level.
Types of Hypothesis Tests
One-sample t-test
Used to compare the mean of a sample to a known population mean.
Independent samples t-test
Compares the means of two independent groups to determine if there is a significant difference between them.
Paired samples t-test
Compares the means of two related groups, such as before and after measurements.
Chi-square test
Used to test the association between categorical variables.
ANOVA
Analysis of Variance (ANOVA) is used to compare means across multiple groups.
Understanding p-value
Definition of p-value
The p-value represents the likelihood of obtaining outcomes as unusual as the observed data, under the assumption that the null hypothesis holds true.
Significance of p-value in hypothesis testing
A smaller p-value indicates stronger evidence against the null hypothesis, leading to its rejection.
Type I and Type II Errors
Explanation of Type I error
Type I error occurs when we reject the null hypothesis when it is actually true, also known as a false positive.
Explanation of Type II error
Type II error occurs when we fail to reject the null hypothesis when it is actually false, also known as a false negative.
Balancing Type I and Type II errors
There is a trade-off between Type I and Type II errors, and the choice of significance level impacts their likelihood.
Confidence Intervals
Definition of confidence intervals
A confidence interval is a range of values within which we are confident that the population parameter lies.
Calculation of confidence intervals
Confidence intervals are calculated based on the sample statistics and the desired level of confidence.
Interpretation of confidence intervals
We interpret confidence intervals as a measure of the precision of our estimates.
Common Misinterpretations
Misconceptions about hypothesis testing
Common misconceptions include misinterpreting p-values and failing to consider effect size.
Avoiding common pitfalls
Understanding the nuances of hypothesis testing helps avoid misinterpretations and errors.
Real-World Applications
Examples of inferential statistics in various fields
Inferential statistics are used in healthcare, economics, psychology, and many other disciplines to make evidence-based decisions.
Importance of inferential statistics in decision-making
Data-driven decision-making relies on accurate interpretation of inferential statistics to inform actions and strategies.
Challenges and Limitations
Addressing challenges in inferential statistics
Challenges include sampling bias, confounding variables, and the assumptions underlying statistical tests.
Limitations of hypothesis testing
Hypothesis testing provides insights but does not prove causation or account for all sources of variability.
Future Trends
Advancements in inferential statistics
Technological innovations and interdisciplinary collaborations are driving advancements in inferential statistics.
Emerging technologies and methodologies
Machine learning, big data analytics, and Bayesian statistics are shaping the future of inferential statistics.
Conclusion
Understanding the fundamentals of inferential statistics and hypothesis testing is indispensable for leveraging data effectively and forecasting trends across diverse populations. These methodologies serve as potent instruments for deriving insights from data and guiding decision-making processes. By enrolling in the best data analytics course in Jaipur and all cities in India, individuals can gain a comprehensive understanding of inferential statistics, empowering them to make informed choices based on data analysis. Armed with this knowledge, participants can contribute meaningfully to advancements in various fields by applying statistical principles to real-world scenarios. Whether in healthcare, finance, or marketing, proficiency in inferential statistics enables professionals to extract valuable insights from data, driving innovation and strategic decision-making. With The Best Data Analytics Course in Jaipur, Agra, Gurgaon, Delhi, Noida, and all cities in India, individuals can unlock the full potential of data analytics and propel their careers to new heights.
FAQs
1. What is the difference between descriptive and inferential statistics?
   Descriptive statistics summarize and describe the characteristics of a data set, while inferential statistics make inferences and predictions about populations based on sample data.
2. How do you choose the appropriate hypothesis test for your data?
   The choice of hypothesis test depends on the research question, the type of data, and the experimental design. Consulting with a statistician or using online resources can help determine the most suitable test.
3. What is a Type III error in hypothesis testing?
   A Type III error occurs when the researcher correctly rejects a null hypothesis that is false but for the wrong reason.
4. Why is it important to consider effect size in hypothesis testing?
   Effect size provides information about the magnitude of the difference or relationship between variables, helping to interpret the practical significance of the findings.
5. How can I improve my understanding of inferential statistics?
   Engaging in hands-on practice, seeking guidance from experts, and staying updated on advancements in the field can enhance your understanding of inferential statistics.